Thank you very much to the lovely Feben Alemu for pointing me in the direction of https://pungenerator.org/ as a means of ensuring we never have to go without a brilliant title! With great power comes great responsibility.
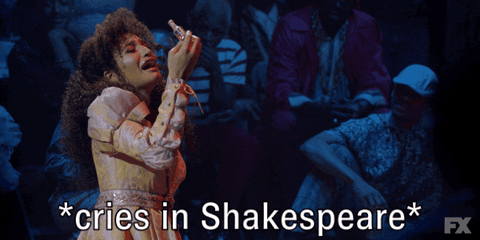
Review
Statistical functionals are any real-valued function of a distribution function
,
. When
is unknown, nonparametric estimation only requires that
belong to a broad class of distribution functions
, typically subject only to mild restrictions such as continuity or existence of specific moments.
For a single independent and identically distributed random sample of size
,
, a statistical functional
is said to belong to the family of expectation functionals if:
takes the form of an expectation of a function
with respect to
,
![Rendered by QuickLaTeX.com \[T(F) = \mathbb{E}_F~ \phi(X_1, …, X_a) \]](https://statisticelle.com/wp-content/ql-cache/quicklatex.com-4b10dae4aa2f3621fc36c4e99fec5e69_l3.png)
is a symmetric kernel of degree
.
A kernel is symmetric if its arguments can be permuted without changing its value. For example, if the degree
,
is symmetric if
.
If
is an expecation functional and the class of distribution functions
is broad enough, an unbiased estimator of
can always be constructed. This estimator is known as a U-statistic and takes the form,
![Rendered by QuickLaTeX.com \[ U_n = \frac{1}{{n \choose a}} \mathop{\sum … \sum} \limits_{1 \leq i_1 < ... < i_a \leq n} \phi(X_{i_1}, ..., X_{i_a})\]](https://statisticelle.com/wp-content/ql-cache/quicklatex.com-718138a55d65f6db2e1c0870b867393a_l3.png)
such that
is the average of
evaluated at all
distinct combinations of size
from
.
For more detail on expectation functionals and their estimators, check out my blog post U-, V-, and Dupree statistics.
Since each
appears in more than one summand of
, the central limit theorem cannot be used to derive the limiting distribution of
as it is the sum of dependent terms. However, clever conditioning arguments can be used to show that
is in fact asymptotically normal with mean
![Rendered by QuickLaTeX.com \[\mathbb{E}_F~ U_n = \theta = T(F)\]](https://statisticelle.com/wp-content/ql-cache/quicklatex.com-03704aa708ac139f338838e18ead98cf_l3.png)
and variance
![Rendered by QuickLaTeX.com \[\text{Var}_F~U_n = \frac{a^2}{n} \sigma_1^{2}\]](https://statisticelle.com/wp-content/ql-cache/quicklatex.com-ea5368a26c9749147451bc9956540db9_l3.png)
where
![Rendered by QuickLaTeX.com \[\sigma_1^{2} = \text{Var}_F \Big[ \mathbb{E}_F [\phi(X_1, …, X_a)|X_1] \Big].\]](https://statisticelle.com/wp-content/ql-cache/quicklatex.com-6bc53efd9d0603e108428da1f5370b7d_l3.png)
The sketch of the proof is as follows:
- Express the variance of
in terms of the covariance of its summands,
![Rendered by QuickLaTeX.com \[\text{Var}_{F}~ U_n = \frac{1}{{n \choose a}^2} \mathop{\sum \sum} \limits_{\substack{1 \leq i_1 < ... < i_{a} \leq n \\ 1 \leq j_1 < ... < j_{a} \leq n}} \text{Cov}\left[\phi(X_{i_1}, ..., X_{i_a}),~ \phi(X_{j_1}, ..., X_{j_a})\right].\]](https://statisticelle.com/wp-content/ql-cache/quicklatex.com-a2c21d5d7f01714fd65d97bc9e5366a0_l3.png)
- Recognize that if two terms share
common elements such that,
![Rendered by QuickLaTeX.com \[ \text{Cov} [\phi(X_1, …, X_c, X_{c+1}, …, X_a), \phi(X_1, …, X_c, X'_{c+1}, …, X'_a)] \]](https://statisticelle.com/wp-content/ql-cache/quicklatex.com-0afd7ad44b3d54edda4f587055601b06_l3.png)
conditioning on their
shared elements will make the two terms independent.
- For
, define
![Rendered by QuickLaTeX.com \[\phi_c(X_1, …, X_c) = \mathbb{E}_F \Big[\phi(X_1, …, X_a) | X_1, …, X_c \Big] \]](https://statisticelle.com/wp-content/ql-cache/quicklatex.com-e5321ca7cbb2cc4e8035f405360737f8_l3.png)
such that
![Rendered by QuickLaTeX.com \[\mathbb{E}_F~ \phi_c(X_1, …, X_c) = \theta = T(F)\]](https://statisticelle.com/wp-content/ql-cache/quicklatex.com-59b21c2f5cd533c3e54b99fef2e75a88_l3.png)
and
![Rendered by QuickLaTeX.com \[\sigma_{c}^2 = \text{Var}_{F}~ \phi_c(X_1, …, X_c).\]](https://statisticelle.com/wp-content/ql-cache/quicklatex.com-5eae440995d70a60fcada6fcb85ecc89_l3.png)
Note that when
,
and
, and when
,
and
.
- Use the law of iterated expecation to demonstrate that
![Rendered by QuickLaTeX.com \[ \sigma^{2}_c = \text{Cov} [\phi(X_1, …, X_c, X_{c+1}, …, X_a), \phi(X_1, …, X_c, X'_{c+1}, …, X'_a)] \]](https://statisticelle.com/wp-content/ql-cache/quicklatex.com-ae2bc59e8a4485598141edc5f81b9a3d_l3.png)
and re-express
as the sum of the
,
![Rendered by QuickLaTeX.com \[ \text{Var}_F~U_n = \frac{1}{{n \choose a}} \sum_{c=1}^{a} {a \choose c}{n-a \choose a-c} \sigma^{2}_c.\]](https://statisticelle.com/wp-content/ql-cache/quicklatex.com-eeb9812359c7a08762e2d186b4ffecfd_l3.png)
Recognizing that the first variance term dominates for large
, approximate
as
![Rendered by QuickLaTeX.com \[\text{Var}_F~U_n \sim \frac{a^2}{n} \sigma^{2}_1.\]](https://statisticelle.com/wp-content/ql-cache/quicklatex.com-2561ada44c52b7270d503a3b65cc4bdb_l3.png)
- Identify a surrogate
that has the same mean and variance as
but is the sum of independent terms,
![Rendered by QuickLaTeX.com \[ U_n^{*} = \sum_{i=1}^{n} \mathbb{E}_F [U_n - \theta|X_i] \]](https://statisticelle.com/wp-content/ql-cache/quicklatex.com-6279990329ef07296dfeb6bf7e3ff6ea_l3.png)
so that the central limit may be used to show
![Rendered by QuickLaTeX.com \[ \sqrt{n} U_n^{*} \rightarrow N(0, a^2 \sigma_1^2).\]](https://statisticelle.com/wp-content/ql-cache/quicklatex.com-a5374b23424474a55f28fc80a9eca29d_l3.png)
- Demonstrate that
and
converge in probability,
![Rendered by QuickLaTeX.com \[ \sqrt{n} \Big((U_n - \theta) - U_n^{*}\Big) \stackrel{P}{\rightarrow} 0 \]](https://statisticelle.com/wp-content/ql-cache/quicklatex.com-03f17552990655c5c7bc7b2446eb452f_l3.png)
and thus have the same limiting distribution so that
![Rendered by QuickLaTeX.com \[\sqrt{n} (U_n - \theta) \rightarrow N(0, a^2 \sigma_1^2).\]](https://statisticelle.com/wp-content/ql-cache/quicklatex.com-9e47c6463a7f5a734945b178bfc8dfad_l3.png)
For a walkthrough derivation of the limiting distribution of
for a single sample, check out my blog post Getting to know U: the asymptotic distribution of a single U-statistic.
This blog post aims to provide an overview of the extension of kernels, expectation functionals, and the definition and distribution of U-statistics to multiple independent samples, with particular focus on the common two-sample scenario.
Continue reading Much Two U About Nothing: Extension of U-statistics to multiple independent samples